Google’s recent algorithm updates emphasize the importance of originality, insight, and unique perspectives in your content. Bernard Huang, Founder of Clearscope, a content-first SEO platform, joined Dana recently to discuss how information gain is your secret weapon to standing out in a sea of AI-generated answers and repetitive content.
Watch the video below and read the recap to learn from Bernard about why information gain is crucial to your SEO and how implementing it into your strategy can protect your site’s organic search visibility, and make your content more engaging.
What is Information gain?
The concept of information gain has been very elusive and hard to parse out given the complexity of how Google works. According to Google’s Patent, “A score for a given document is indicative of additional information that is included in the document beyond information contained in documents that were previously viewed by the user.”
Information gain is content that covers concepts and entities on the fringe of Google’s Knowledge Graph for the topic.
“Information gain is the idea that when users browse the internet for any given topic, that they want to read more about what the topic is directionally heading towards, and that’s based off of course, what Google is going to be serving the user.” Bernard Huang
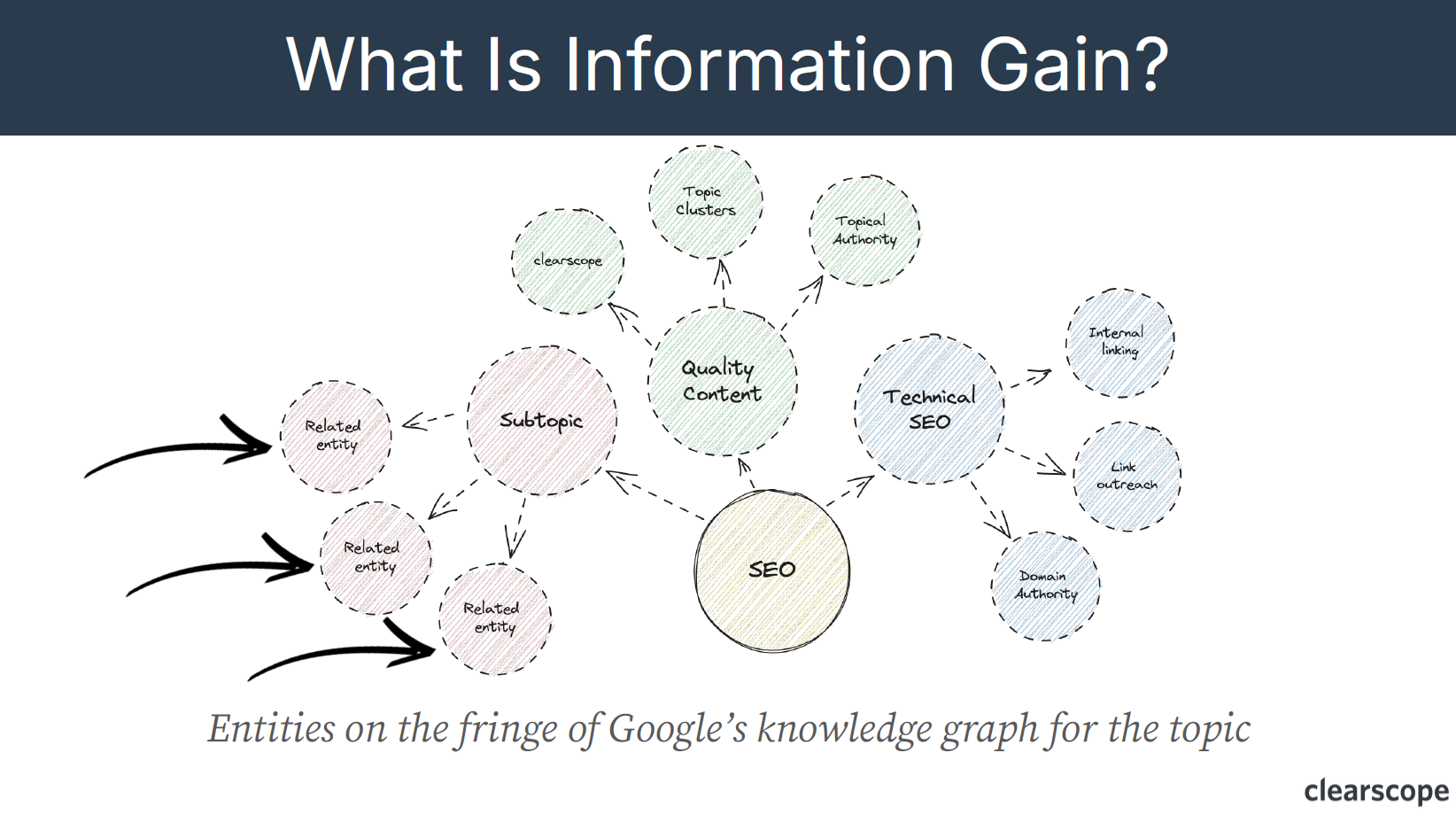
Using the chart above as an example to explain how information gain works, if a user is searching “what is SEO?” and Google provided only three results on page one that all repeated the same content to say: SEO is about Technical, Quality Content, Authority, Brand, and so on. This would be a very poor search experience for the user who would be displeased with the results.
“Google wants to serve an authoritative result for rank number one that covers the basics of what SEO is. But then they might look at number two and say, okay, let’s make sure that this document adds something to the topic for the user. So if result number one really talks about quality content and technical SEO, then Google would assign a score of, something positive if rank number two were to start talking about, say, artificial intelligence (AI) or Large Language Models (LLMs). In that particular case, we can then say that, the second document, which is now talking about AI, LLMs, etc, are now being brought to this particular topic.” Bernard Huang
You can see that highlighted in the chart above as depicted by the “Subtopic” diagram. Google has a strong understanding of how topics relate to one another—in Google this is known as the Knowledge Graph—and then refers to entities on the fringe of that particular topic. So in the example of “What is SEO?” the entities (subtopics) that are on the fringe would be the LLMs, AI, Gemini, GPT etc.
Why information gain matters to Google
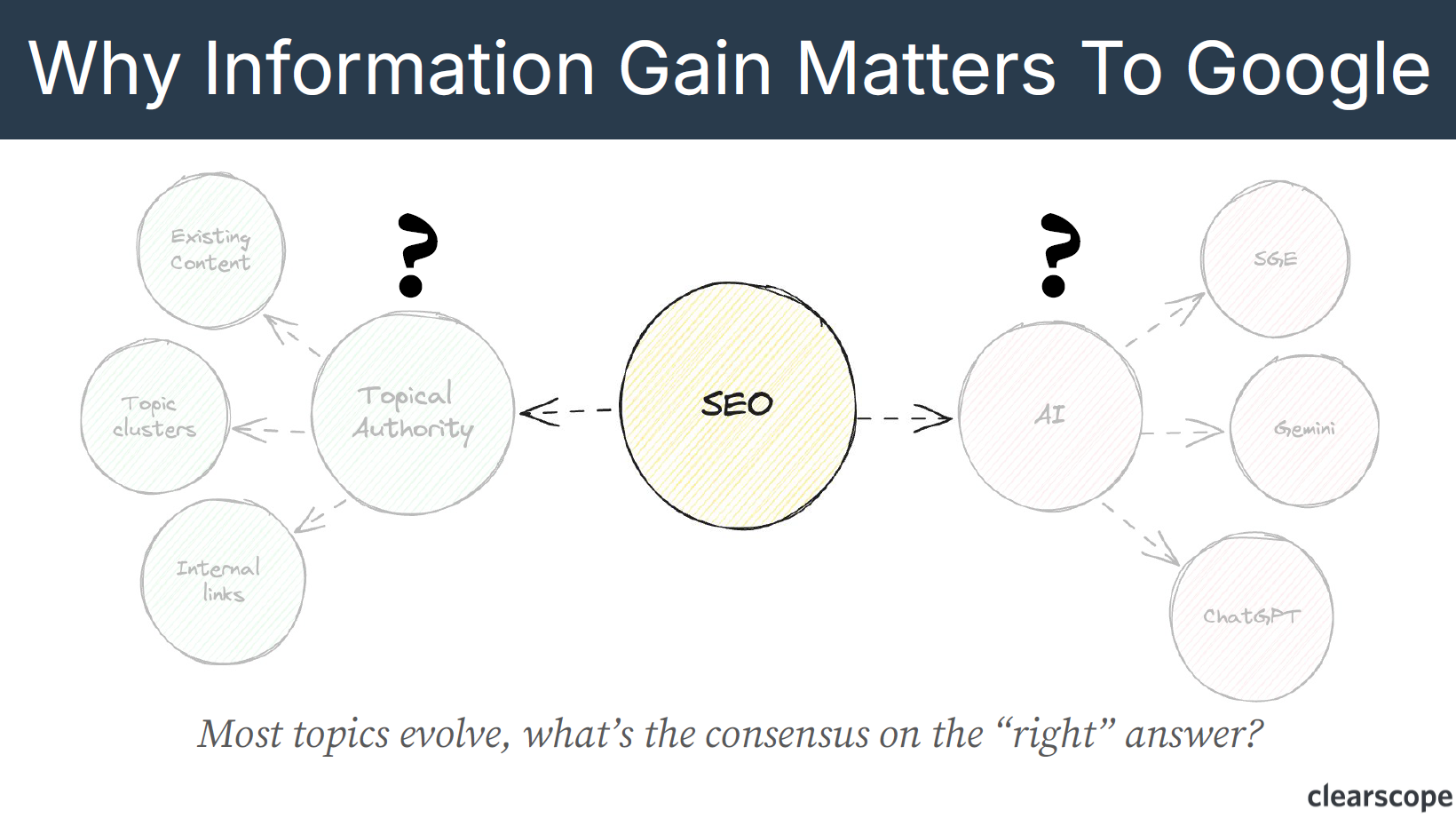
Most topics evolve and Google wants to give users the best answers to their questions.
“SEO is evolving towards more AI, or evolving towards more topical or brand authority. Google needs to be aware that is happening, and so they can serve documents that give those answers to the questions or searches that users are performing. Pretty simple.” Bernard Huang
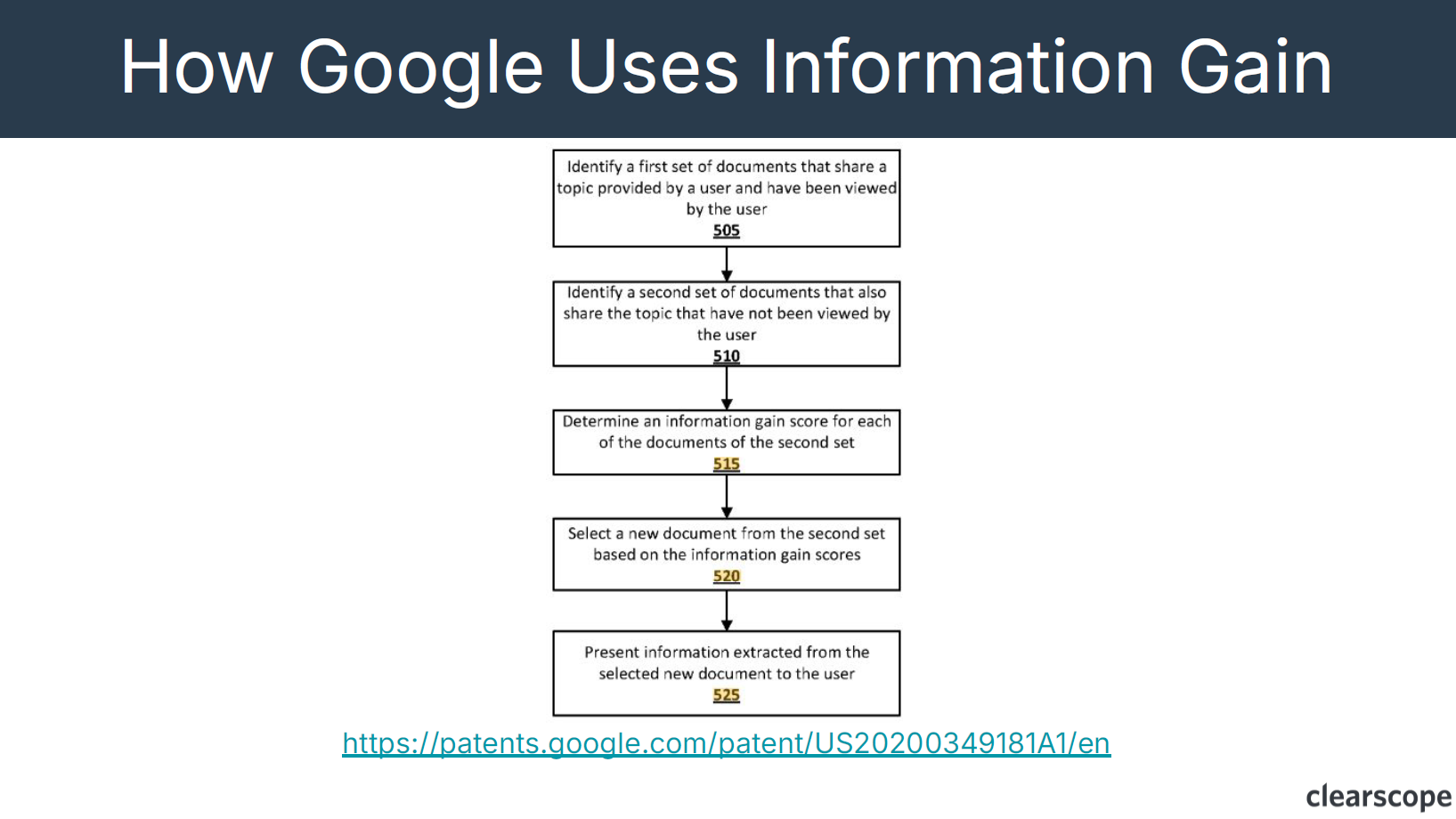
Based on the Google Patent you can see how Google uses information gain to look at documents alongside other documents.
💡 Document refers to whatever the search result has returned, so it could be a website landing page, PDF, video, forum thread, and so on. Whatever content Google has returned for a given query in search results.
The process Google performs is:
- Google reviews a set of documents that have been viewed by the user. Google then tries to understand all of the concepts within the document (the “entities”) that have been contributed to the specific topic.
- Then, Google is going to look at other documents that have covered the specific topic and evaluate if they are bringing any new entities to that particular topic in a meaningful way. So did the second set of documents add additional value or provide new information? The more or addition your document brings, the higher the score it receives, Google will then select a new document from the second set based on the score that it has assigned.
🌐 What is an entity in SEO? An entity is a distinct, identifiable concept or thing (like a person, place, or idea) that search engines recognize. It helps search engines understand context and meaning, improving search accuracy through semantic search and knowledge graphs.
Information gain in practice
Google incorporates information gained regularly into SERPs, this is why you see non-stop fluctuations with rankings and positions because Google is constantly running A/B tests. This is how the algorithm is designed to work.
Let’s go back to the “What is SEO?” example, say that Google is testing ranking content talking about “topical authority” in the number one position, and content talking about “artificial intelligence” in the number two position. Google will then swap the results.
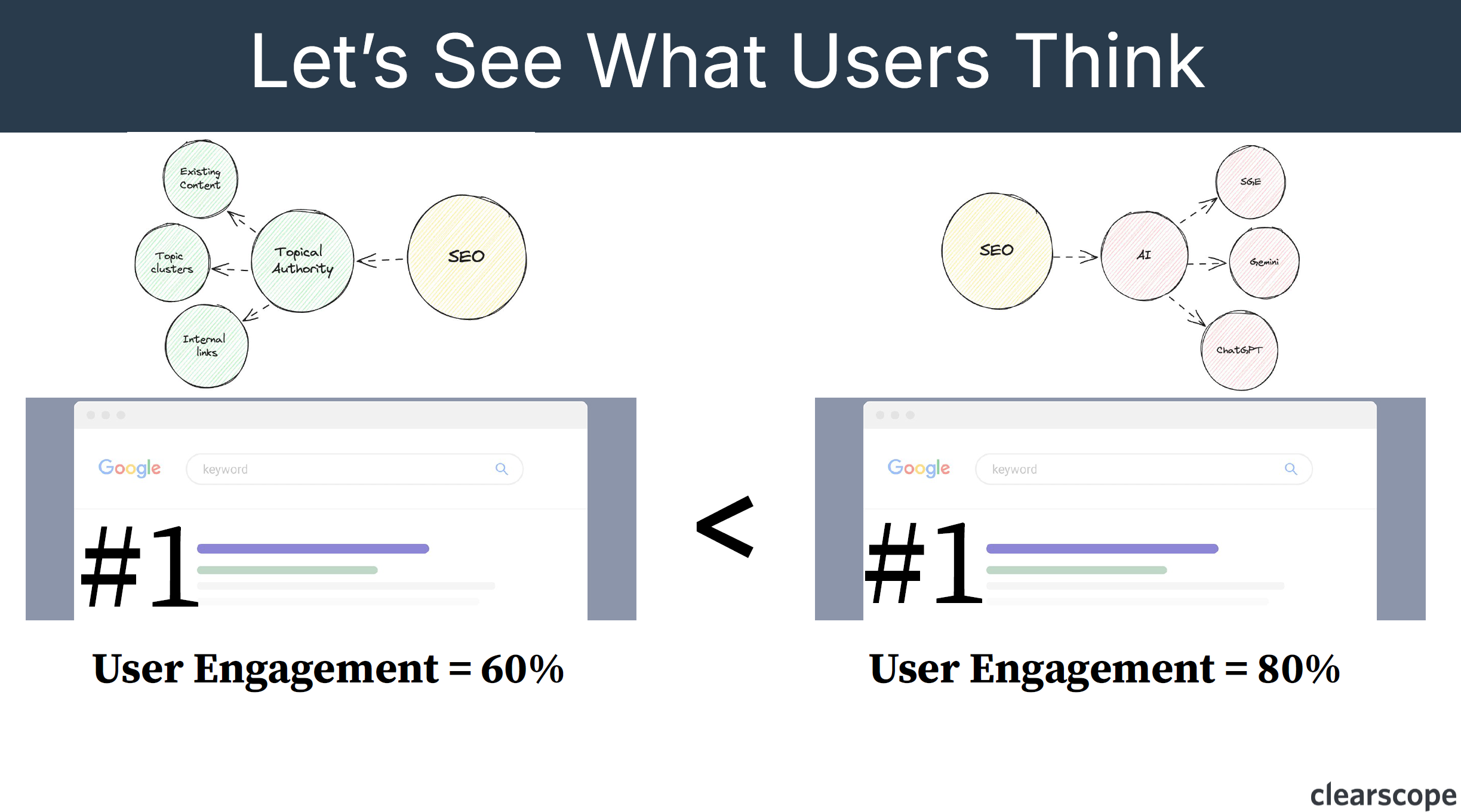
“They’ll say okay if we displayed this result with this lean or direction or additive then you know what the user engagement is like? User engagement is, I think, quantified by the content’s ability to conclude a Search journey or basically somebody lands on your content and they exit out of their search. If they go back to Google, click on some more results, perform additional searches, then that would negatively influence your user engagement score.” Bernard Huang
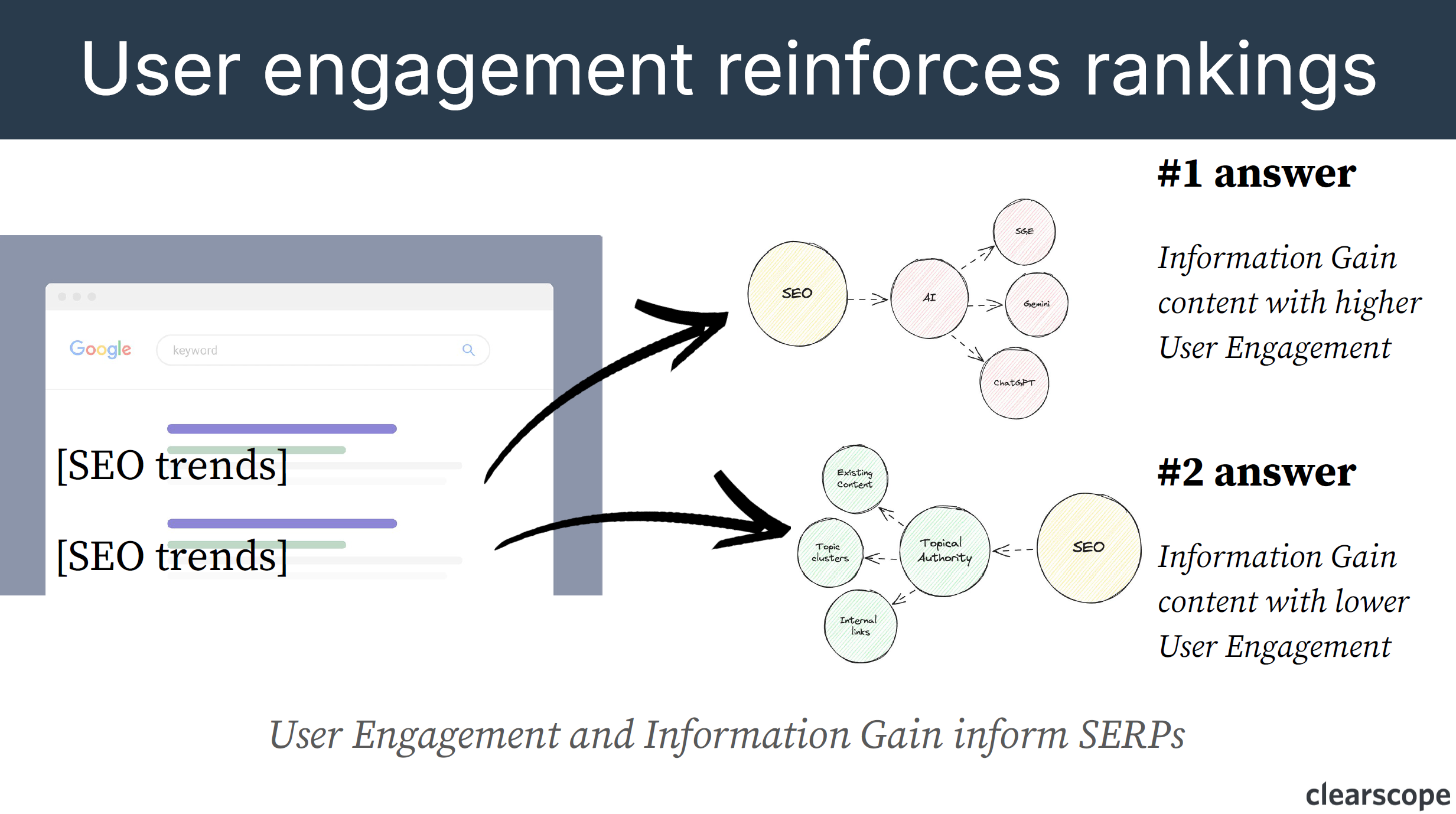
So in the “What is SEO?” example, if user engagement is higher for the AI topic, and the information gained leans towards artificial intelligence, then that will rank as the number one answer.
“Information gained content [with] higher user engagement will outperform information gained content with lower user engagement. Of course, this is from the core documents, but the user engagement is what reinforces the rankings, and Google understands enough about the topic to want to serve a document that gets produced by it, but at the end of the day, the algorithm doesn’t know whether we, as users or searchers of Google care more about artificial intelligence or brand authority, right? Google is just saying we’re impartial, let’s see what users think.” Bernard Huang
Stay in The loop of what's new in digital marketing
The role of large language models (LLMs) in information gain
The concept or theory of Information Gain is becoming more important as a result of the popularity and increased adoption of artificial intelligence (AI), more specifically the influence that Large Language Models (LLMs) have had on the digital marketing ecosystem.
How do LLMs work?
On the surface Large Language Models (LLMs) are sophisticated autocompletes. You can see in the image above a model that breaks down how ChatGPT2 works. Essentially words follow words, sentences follow sentences, and paragraphs follow paragraphs.
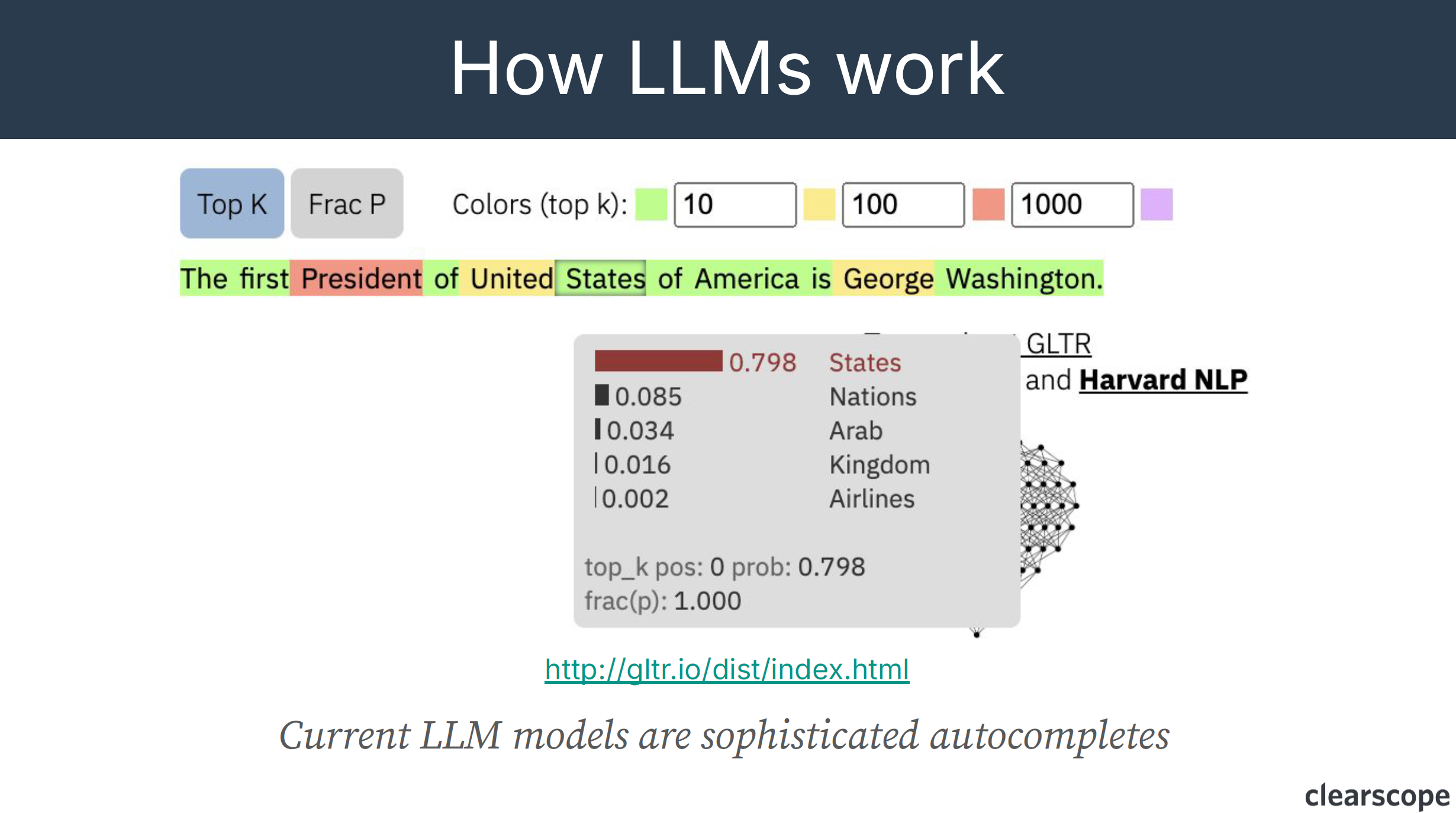
“I think [it’s] really important to emphasize the sophisticated autocomplete part of LLMs, because I think that this is where calling an LLM AI is not the right phrasing for it, it’s not intelligent in that way. I wonder if people who don’t use, or [aren’t] versed in LLM research or anything may think that it’s actually like thinking. But the reality is, it’s just what they think the next word is going to be, with some certainty. And even the newer version of ChatGPT, even the one where it’s oh here’s their thought process, but you can’t look into it too deeply, it’s still just, fancy autocompletes. We’re not intelligent yet. At least not for what the consumer has access to. Maybe there’s some fancy actual AI somewhere in the Google mothership that normal people can’t look at but…” Dana DiTomaso
In the example you see in the image above you have:
- States with an 80% likelihood of following United given the first president of United.
- Nations with an 8.5% percent chance.
- Arab with a 3.4% chance.
- Kingdom with a 1.6% chance.
- And Airlines with a 0.2% chance.
United Nations, United Arab, United Kingdom, United Airlines are all possibilities, but then given George Washington and America, that may influence the LLM towards States.
ChatGPT has been such a revolution because LLM outputs for ad writing and short-form content, based on highly trained data sources, are actually really good, with a correct output rate of 95%. Small inconsistencies in short writing result in only minor discrepancies.
“Where we start to see some holes and some gaps are then within limited training data. If you have a sentence that’s only 90% correct to start, over the course of five sentences, you actually end up with a 59% accurate paragraph. And you can imagine that starts to look very questionable. Newer topics, aka , this is not going to be as good because there’s less training data on it. Also, one of the big caveats is long form content. And that’s to say that, if we have paragraphs that are 95% accurate, given the 99% on a sentence over five sentences, get you a 95% accurate paragraph, you can imagine over 77% accurate essay. The longer it goes, the more that it has to work off of what it already presented. And then, the accuracy continues to dwindle.” Bernard Huang
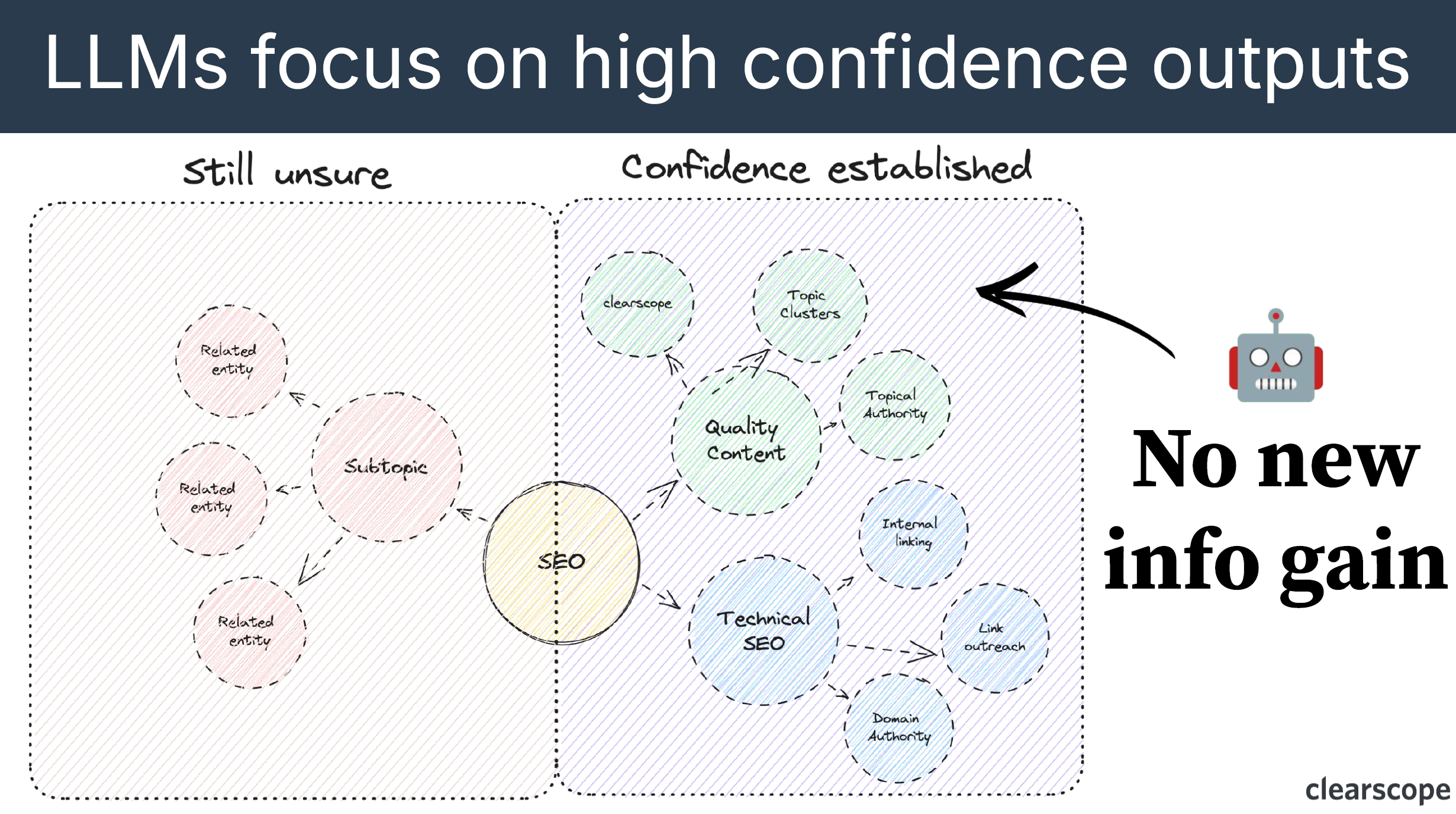
LLMs focus on high confidence outputs. Going back to the Knowledge Graph example, “What is SEO?”, LLMs strongly prefer the things they are confident in because that prevents inaccuracies, hallucinations, and some biases.
“Obviously, this is where the concept of temperature comes into play. So if you have dabbled around with a lot of LLMs and you’re going into the nitty gritty, you’ll have noticed that there is this idea of temperature. Temperature just gives the model more leniency to venture outside of the bounds of what it thinks is likely to be right. If you dial up the temperature, then you start to get things that the LLM will suggest, like gluing cheese to pizza, which we’ll come back to in a second. Anyways, all of that to say that LLMs are basically designed as, you know, stock LLMs. Obviously, there’s a lot of things where we’re trying to improve LLMs within certain niches and certain applications, but on average, LLMs are designed to focus on being not wrong. And not wrong means in this particular case means no new information being gained.” Bernard Huang
LLMs struggle with information gained because their primary focus is on topics with a high confidence of being related to the core topic you’re writing about. Information gain is still human directed, which means that we need to come in and add our perspectives, our first party data, and experiences to make the content useful and unique.
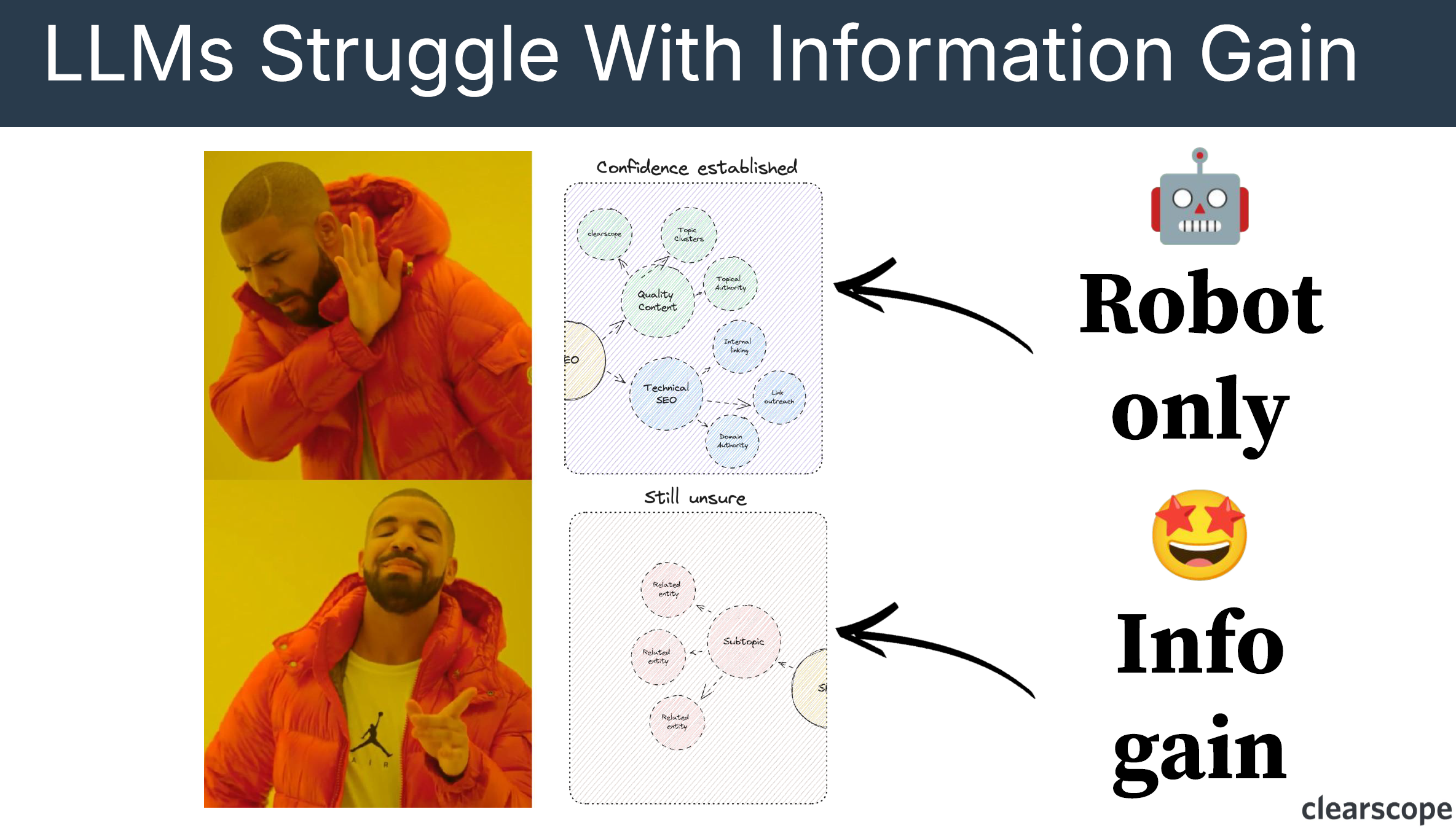
Google evaluates content for redundancy
Putting this all together, going back to the patent (Section [0050]), we can see that according to the Google Patent they are looking for document redundancy.
“As they view consecutive documents returned in response to a search query, they may be asked (e.g., via a web browser plugin), for each new document they consume, a question such as “Was this document/information helpful in view of what you’ve already read?” or “Was this document redundant?”
“You can imagine if two documents say the same thing, then, one of them doesn’t need to exist in the SERP. I think, in the past, this would have been plagiarism or Panda, but natural language processing and machine learning has evolved quite a bit. And now it’s no longer about just exact word for word copies. It’s actually more of an entity specific redundancy, right? If I say SEO is quality content, authority backlinks, technical SEO, those are entities.
How I say it. It doesn’t matter, right? As long as the natural language processing understands that those entities are what I’m contributing to the topic, I can say it however I want, and if another document says all of that as well, my document is essentially redundant because it’s not saying anything new.” Bernard Huang
Google and LLM evolution
So how can you prevent inaccuracies and actually gain information? We know that topical relevance fluctuates over time. The one caveat to this is if you’re writing about facts and history or things that don’t change like static concepts.
LLMs require constant training to understand how humans who contribute to the internet think about certain topics and some topics change faster than others. An example of a topic rapidly changing would be AI or US politics during election season. Topical relevance is going to fluctuate based on the topic at hand and Google needs to train its understanding of topics quicker and better.
Before ChatGPT, Google had a monopoly in search, which allowed them to dominate without much competition which has shaped how SEO content was created. Decades of algorithm updates and suggested best practices have pigeonholed SEO content creators into rigid templates, resulting in an “existential crisis” for Google as much of the content optimized and created for search engines is low-quality and lacks true value. This brings us to the Reddit deal, Google needs a direct data source to help inform topical evolution, and Reddit has authentic users who are contributing and discussing any given topic. With access to Reddit’s content, Google can now train their artificial intelligence.
“With the Google API leak the UGC (User Generated Content) discussion effort score has been boosted quite significantly. And obviously we see a lot of Reddit, Quora, and different forums showing up. And that’s, I think, Google saying, yeah, we’ve scanned all of these documents that could rank for any given topic.
And that for each of those documents is not great, but discussions, user generated content is actually pretty good. So let’s just boost that signal over there, and then, hopefully, I think this is more theory and propaganda, but hopefully we’ll start to incentivize content creators to produce perspective led, experience rich content. And that’s our goal. So Google needs more, more trusted sources. You can see here that theoretically, this is what I imagine, how Google is taking the The discussions on Reddit and using it to inform, where a topic is evolving.” Bernard Huang
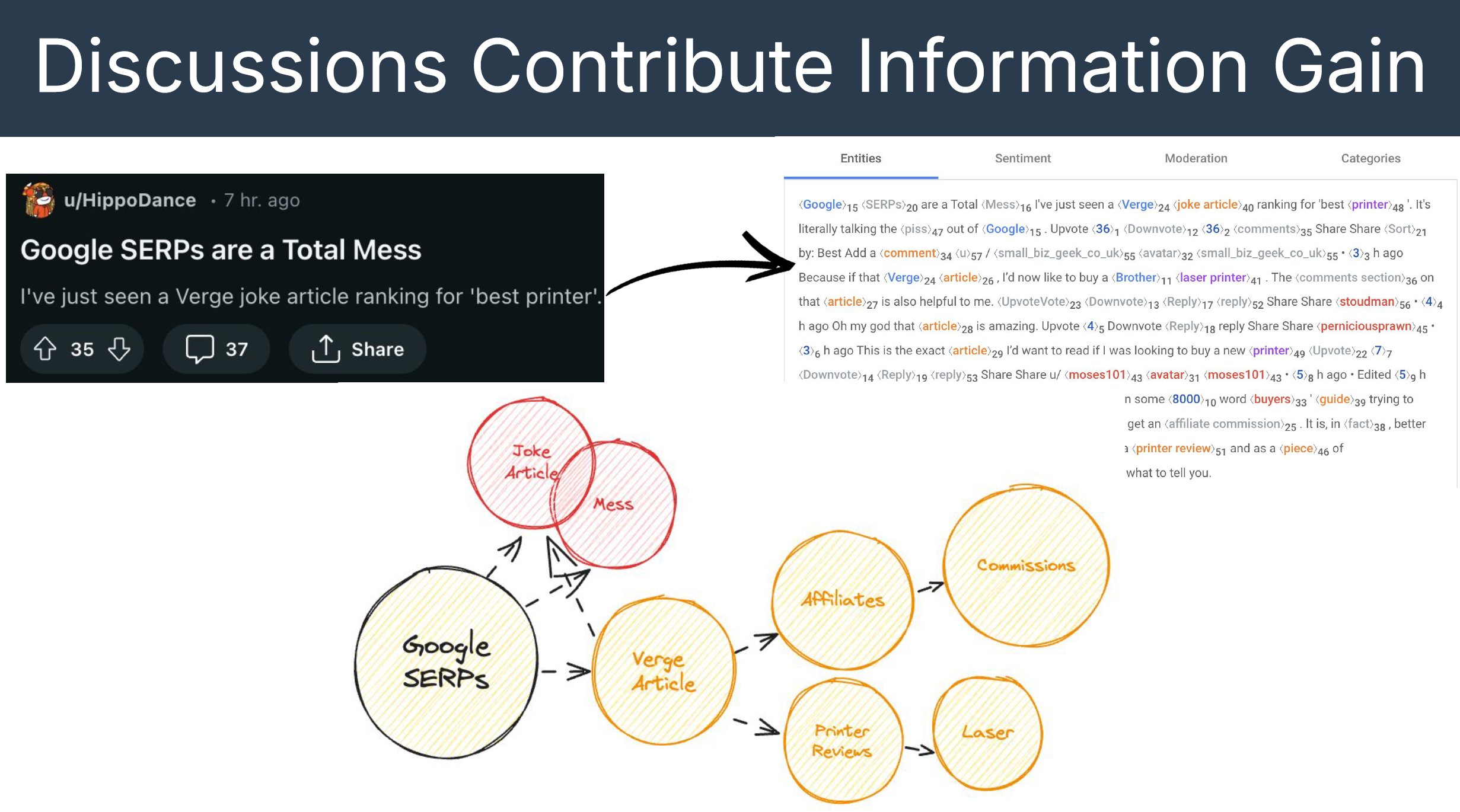
In the diagram above, you can see that Bernard suspects Google now has a direct data feed from Reddit. Once comments and posts are published, Google automatically ingests and analyzes the content through its natural language processor (as shown on the right side of diagram above). This allows Google to understand the core entities or concepts that are evolving around a particular topic. From there, Google can build out loose associations within its Knowledge Graphs.
Using Bernard’s example of the “Google SERPs are a Total Mess” Reddit post, Using Bernard’s example of the “Google SERPs are a Total Mess” Reddit post, Google could theoretically use this to build out topics like “Google SERPs are a joke,” “they are biased towards publishers,” or “affiliates are getting screwed,” and so on. UGC (User-Generated Content) sources also come with built-in trust signals, which Google can weigh more heavily when determining what matters and what doesn’t.
Then LLMs like OpenAI (ChatGPT) join the conversation saying “we need this too”. Their outputs are only as good as their inputs so they need training data from UGC sources and publishers which leads to them creating partnerships with Reddit and other platforms.
Is Reddit Really a Reliable Source of Information?
“It’s wild to me that the internet is basically turning into a Reddit machine. Like I have to say, I don’t think that’s necessarily the best source of content. Having been a longtime Redditor myself. Generally Reddit is a cesspool and it’s also very North American biased. And I feel like it’s just reinforcing the internet’s already biased towards North America, specifically US stuff. And then Reddit’s mostly Americans. And it’s just like this feedback loop of American content.” Dana DiTomaso
“I don’t think this is long term good, like just for either Reddit or Google or OpenAI. I think Reddit itself is, I think Interesting, because, like I was remarking, I think that communities are the antithesis of monetization.
And I think that as we see Reddit become polluted, If you will, because of the rise of visibility that Google is giving it that a lot of Redditors are saying this just sucks. Let me go to another platform. Good news is that there aren’t that many currently that exist, like alternatives. We’re not seeing the dramatic decline of UGC, but I think it’s likely happening, and if that is the case, then, the well of information, again, it’s like questionable information that Reddit contributes is going to dry up for Google and OpenAI and other language models that tap it.” Bernard Huang
The Problem with The Data
All LLMs need better training data and this comes back to the fact that topics are always influx. If you ask what the price of Bitcoin is you need to know the price at the exact moment in time, not what the price was at the beginning of the year.
Every LLM is trying to find ways to look more informed and less stupid which is why they want the training data and information gain. The problems that arise from this, however, is the use of smaller sample sizes to inform the direction of where a topic could head, this leads to mistakes (ie. The Google AI overview recommending glue on pizza) or trusting non-authoritative sources as sources of truth (ie. drinking urine example for kidney stone passing recommendations).
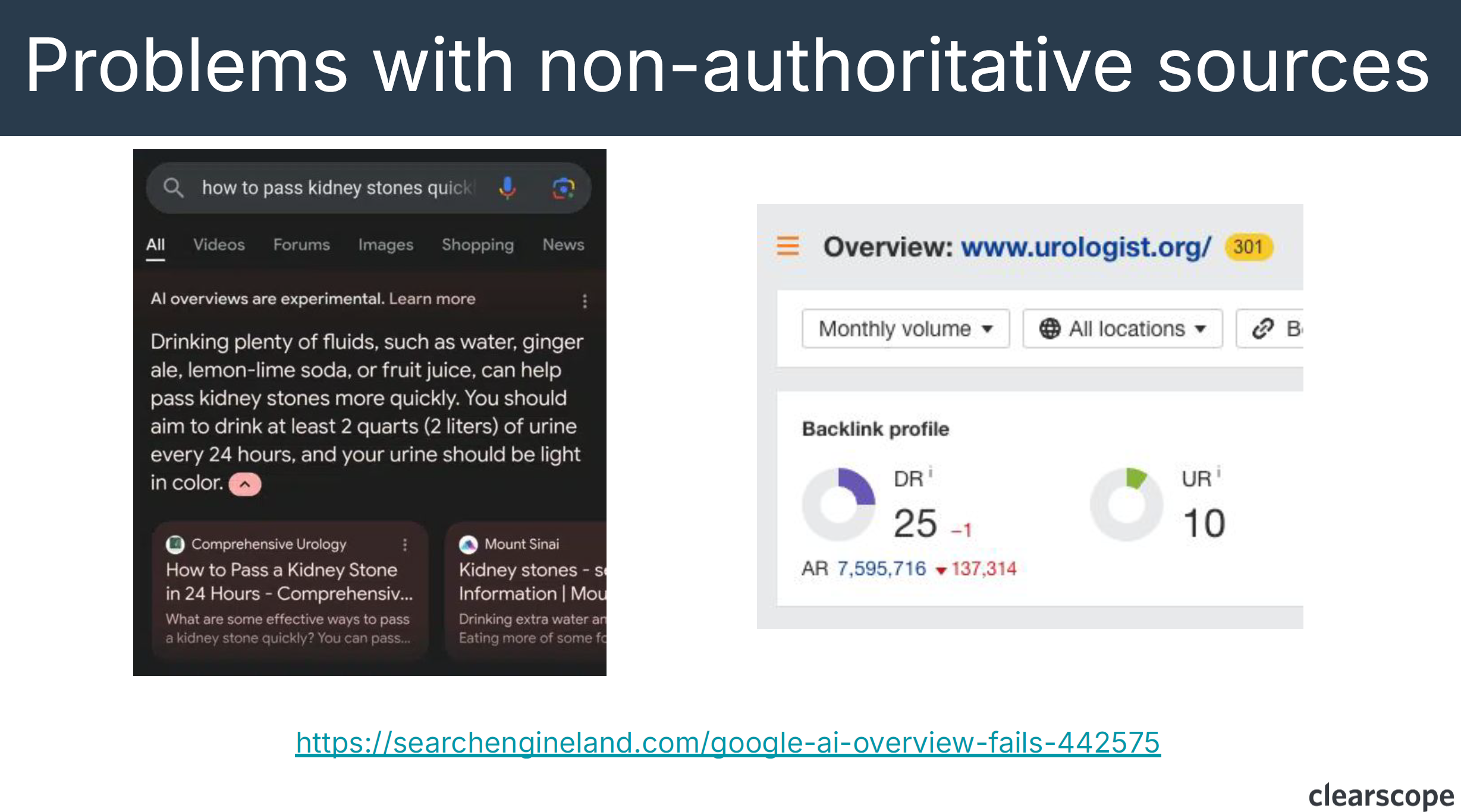
Never miss expert insights and tips!
Google AI overviews and entities
Taking a deeper look into application, Bernard runs through an analysis he completed on AI overviews and entities.
First, he performs a Google search on “What is UGC” and gets an AI overview result explaining his query. He then takes this text and pastes it into Google Cloud for natural language processing (entity recognition). You can see from the results (see image below) that Google identifies UGC as “content”, a blog post as a “work of art”, and Burberry as an “organization”. AI overviews are entity dense responses.
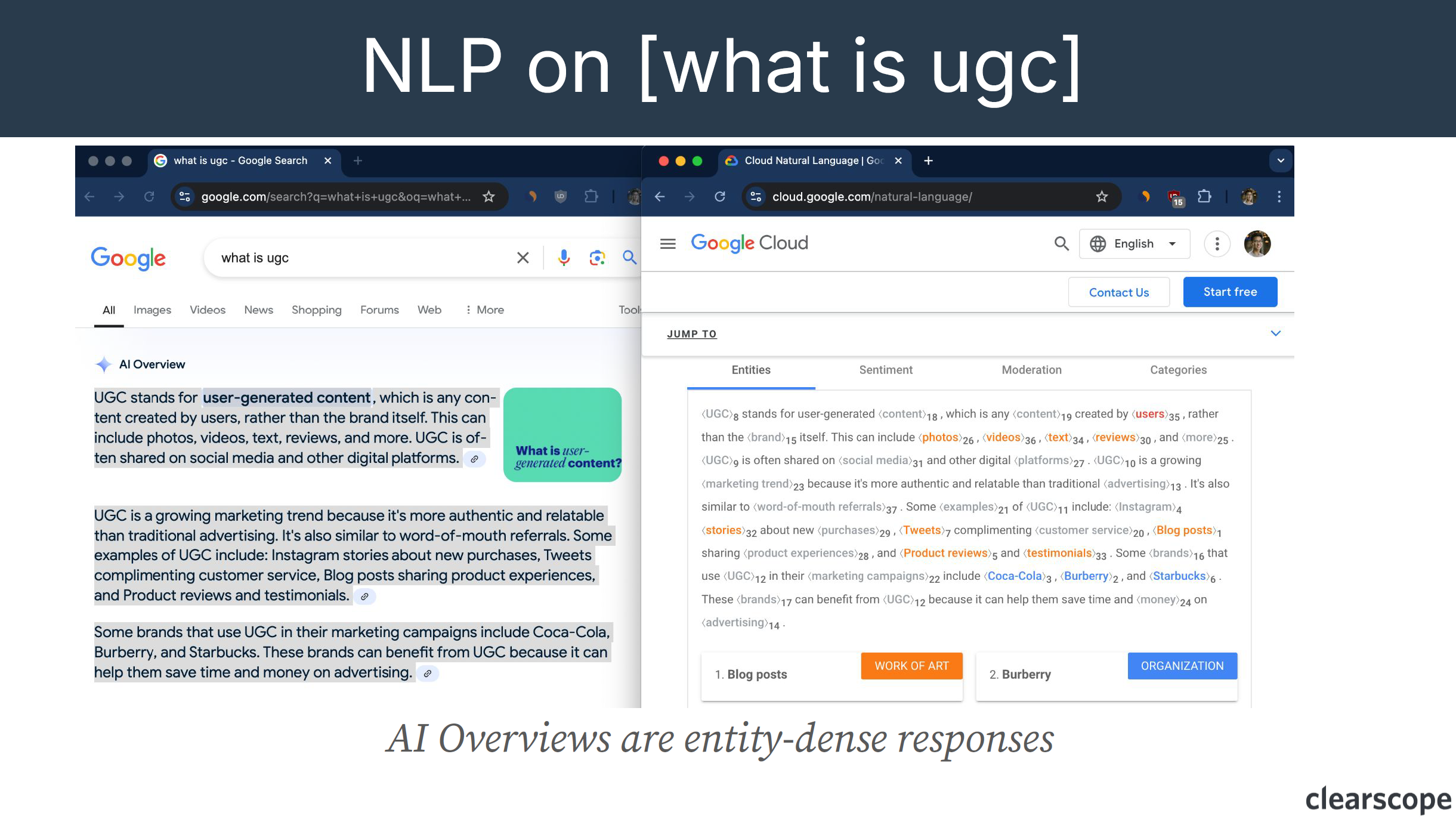
In this UGC AI overview example the response was 113 words of which 37 were entities identified by Google. This makes it 33% entity occurrence (37/113)!
“Looking at content in the SERPs, it’s closer to 10%. This is deduped and all that stuff. So AI overviews are essentially entity rich Knowledge Graphs on the topic that Google understands. So taken a step further, I take the outputs of the different entities that the AI overview has generated that is then processed by Google Natural Language Processing.” Bernard Huang
Bernard Huang further dives into how Google AI Overviews and Natural Language Processing (NLP) generate and map entities from content using category associations, revealing complex relationships between concepts like user-generated content (UGC), product reviews, and brand value. By processing these connections using ChatGPT, he categorized core themes such as social media platforms, brands, and customer experiences.
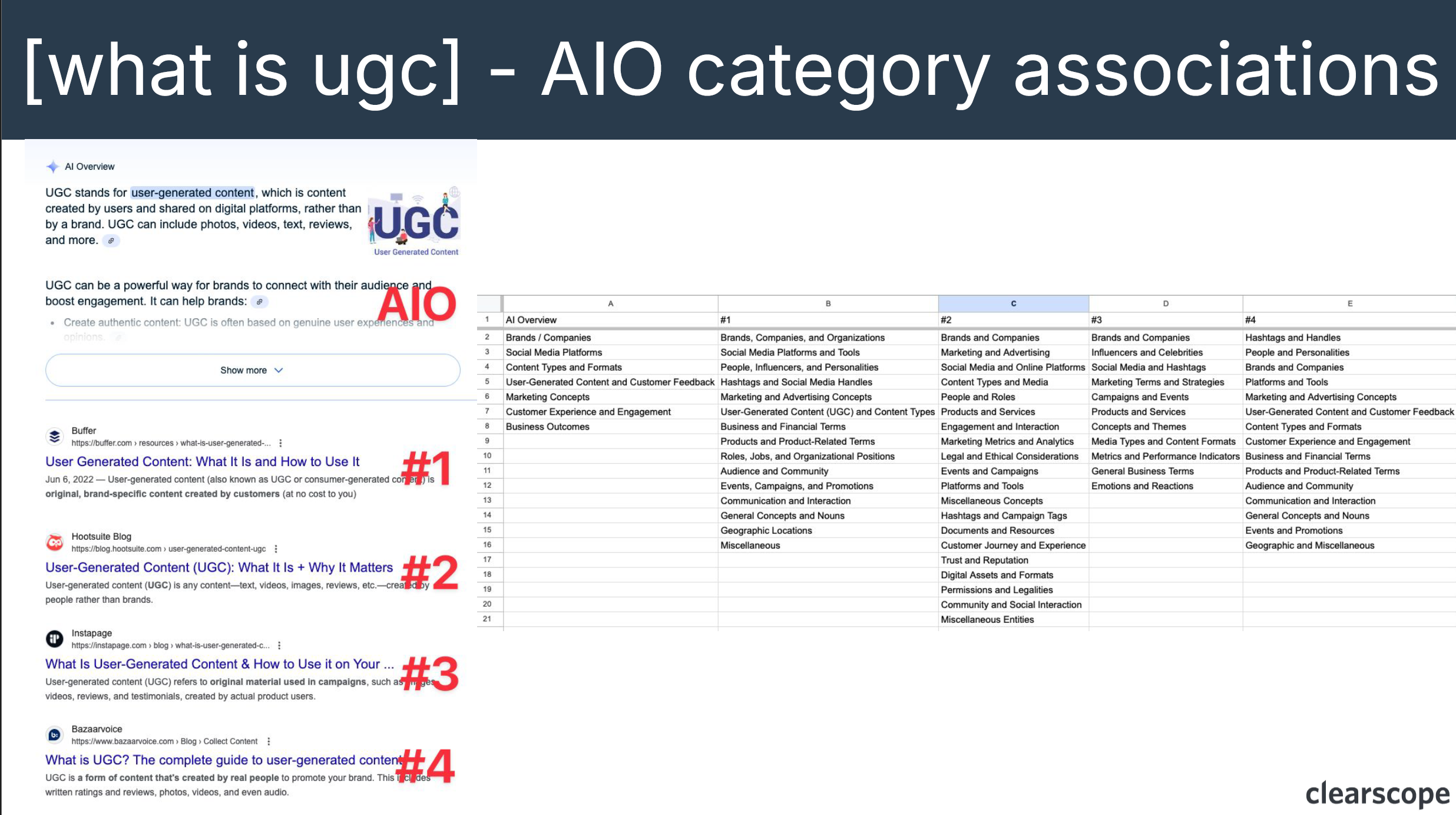
Through entity mapping across multiple articles, Bernard demonstrates how Google’s understanding of UGC content reflects Semantic SEO principles, showing consistent themes across top-ranking results with some variation in specific categories like legal considerations or geographic locations.
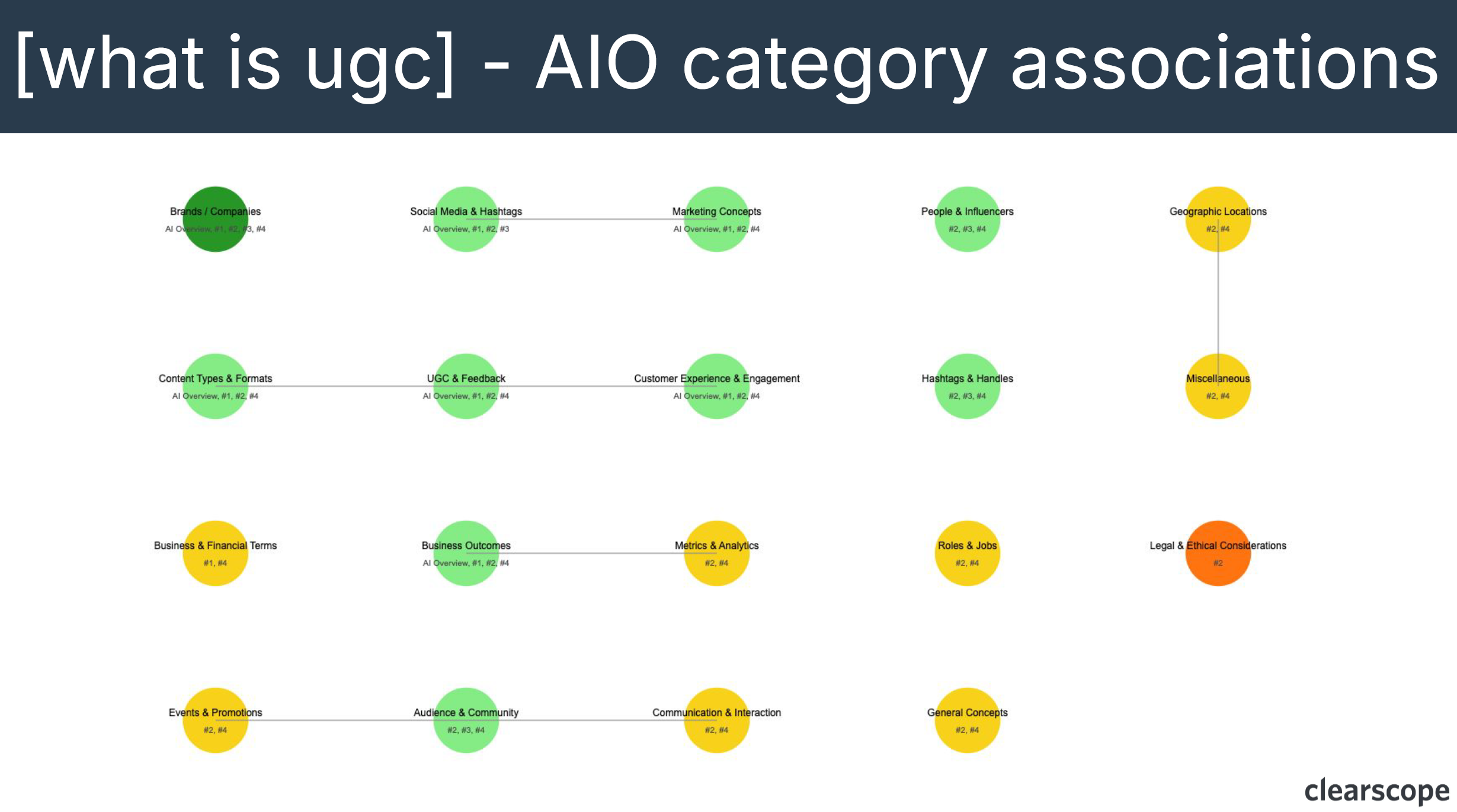
“And Semantic SEO is the fact that Google does indeed care that your content is somewhat relevant to the topic, and that’s why Clearscope as a software exists, right? We give you insights into the core entities that you should discuss for any given topic. And that’s because, to a degree you do need to prove to Google that you do know what you’re talking about.
But, as you can see here, then you need to add to that particular topic with , in different directions that you think the topic could be evolving and people have been calling this first party data and perspectives, experience, that sort of thing. I think it doesn’t particularly seem to matter.
Google is not like, oh my God, I like you for this, you need to talk about legal and ethical considerations, right? As long as you cover the core of what Google expects you to cover, you’ve covered your bases there, and then, yeah, you can do a survey, you can write about your own experience, like implementing a UGC program and how it worked or didn’t. It doesn’t matter just as long as the core concepts are covered and then you’re bringing something new or interesting to the table.” Bernard Huang
Lifecycle of SEO content
Exploring topical evolution using further with real life examples, Bernard narrows in on entities in the How Google Rankings Work data from Mario Fischer.
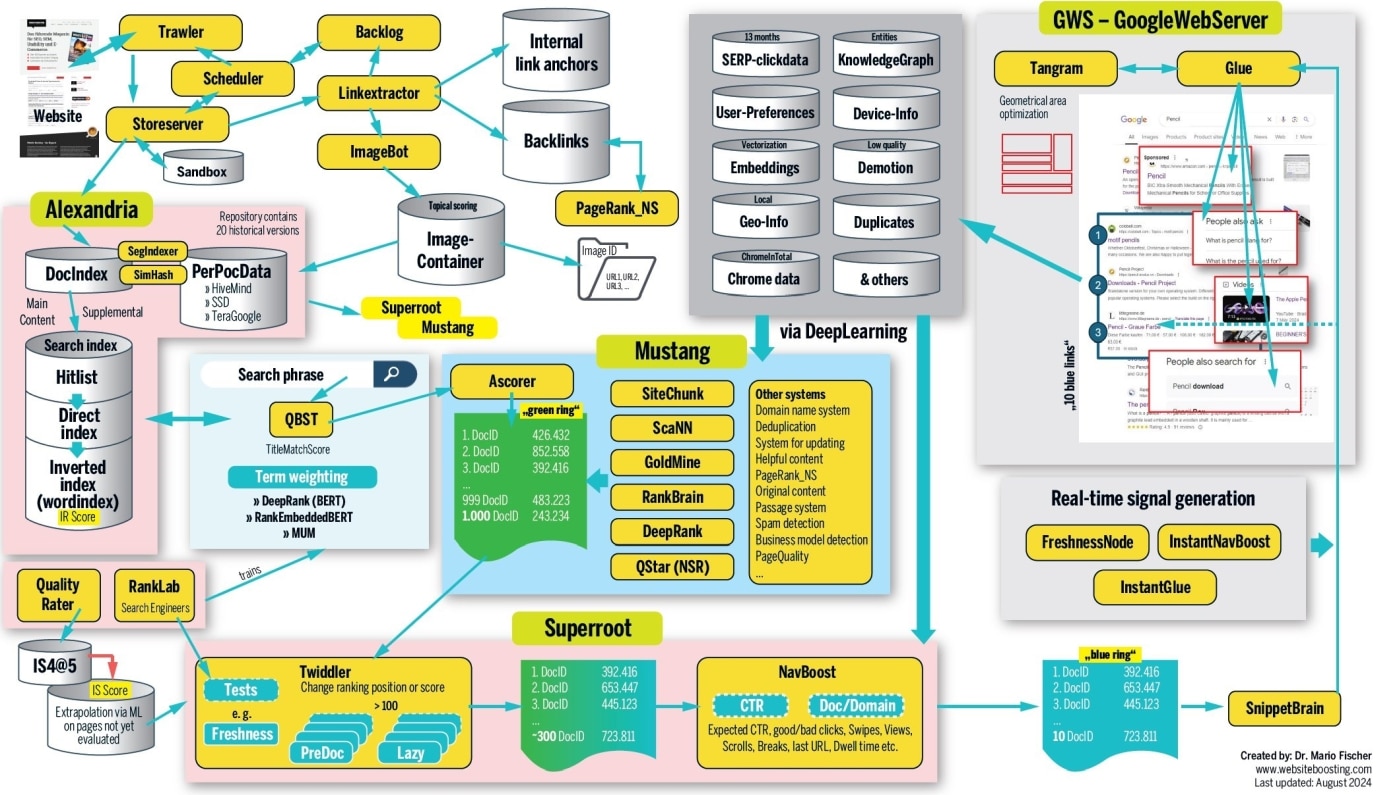
Within the API leak documents, was called out, specifically under “splittingFeatureScore – The of content features when selecting this splitting feature to split the node.”
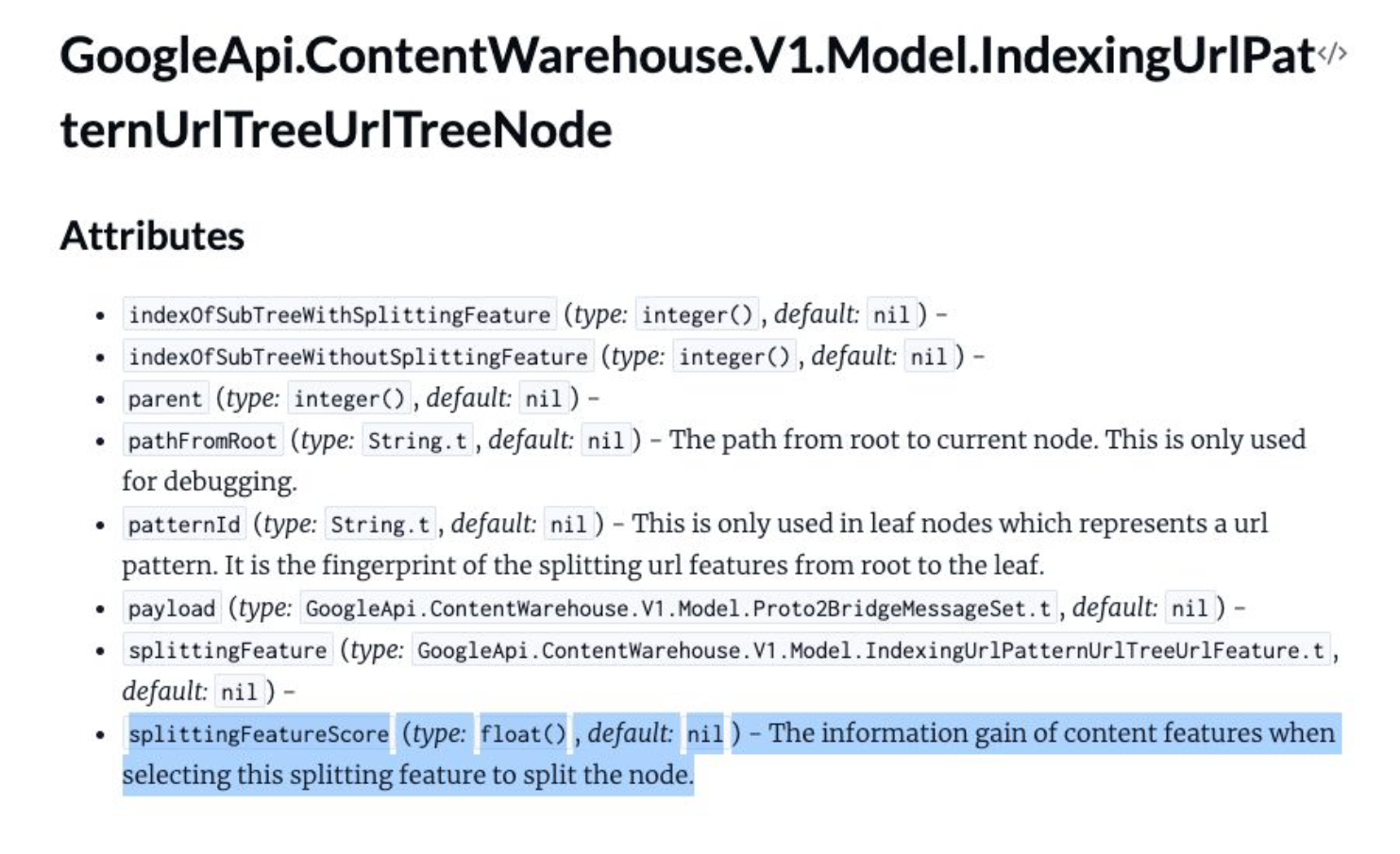
Using the Google Algorithm Leak as an example of SEO content lifecycle, Bernard explains how this works.
SparkToro and iPullRank are the first to break the news on the Google Algorithm leak that was published on May 27, 2024. This content is . Now, part of Google’s first steps is to evaluate the trust signal for the domain(s) (“because not everyone can contribute to swaying Google into believing that cheese needs to be glued on pizza.” Bernard Huang).
Google evaluates different attributes to determine trust signals. These include:
- Page Embedding
- Site Embedding
- Site Focus
- Site Radius
- Version ID (version of the published piece of content)
When Google’s AI Gemini is asked “What topics are iPullRank authoritative in?” It identifies that iPullRank is authoritative in technical SEO, content strategy, and SEO content. This makes sense because they are an agency. Taking this back to the Google attributes used to evaluate trust signals of site embedding, site focus score, page embeddings, and site radius, Google evaluates the content relevance and authority—in this case the algorithm leak, through page embedding. Google calculates how closely the topic aligns with the overall site’s focus, determining if the content qualifies as relevant. If it does, Google tests it in search results, potentially evolving its understanding of the topic as it gets indexed and ranked.
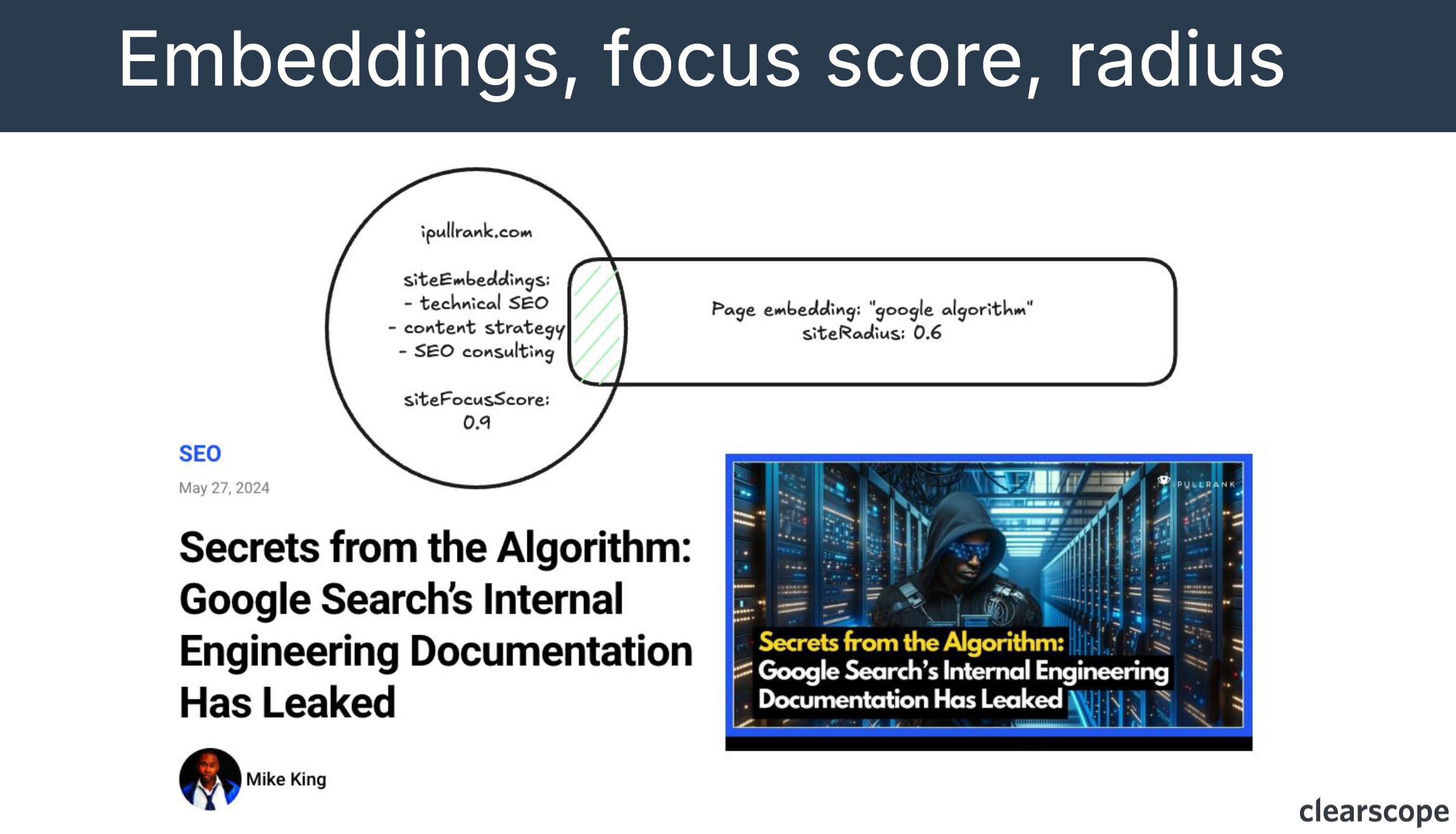
“Alright, so then user interaction informs the algorithm. When Google displayed that iPullRank result at position 1, were people finding what they needed? Reads, clicks, scrolls, dwells, whatever. So yeah, it looks pretty good.” Bernard Huang
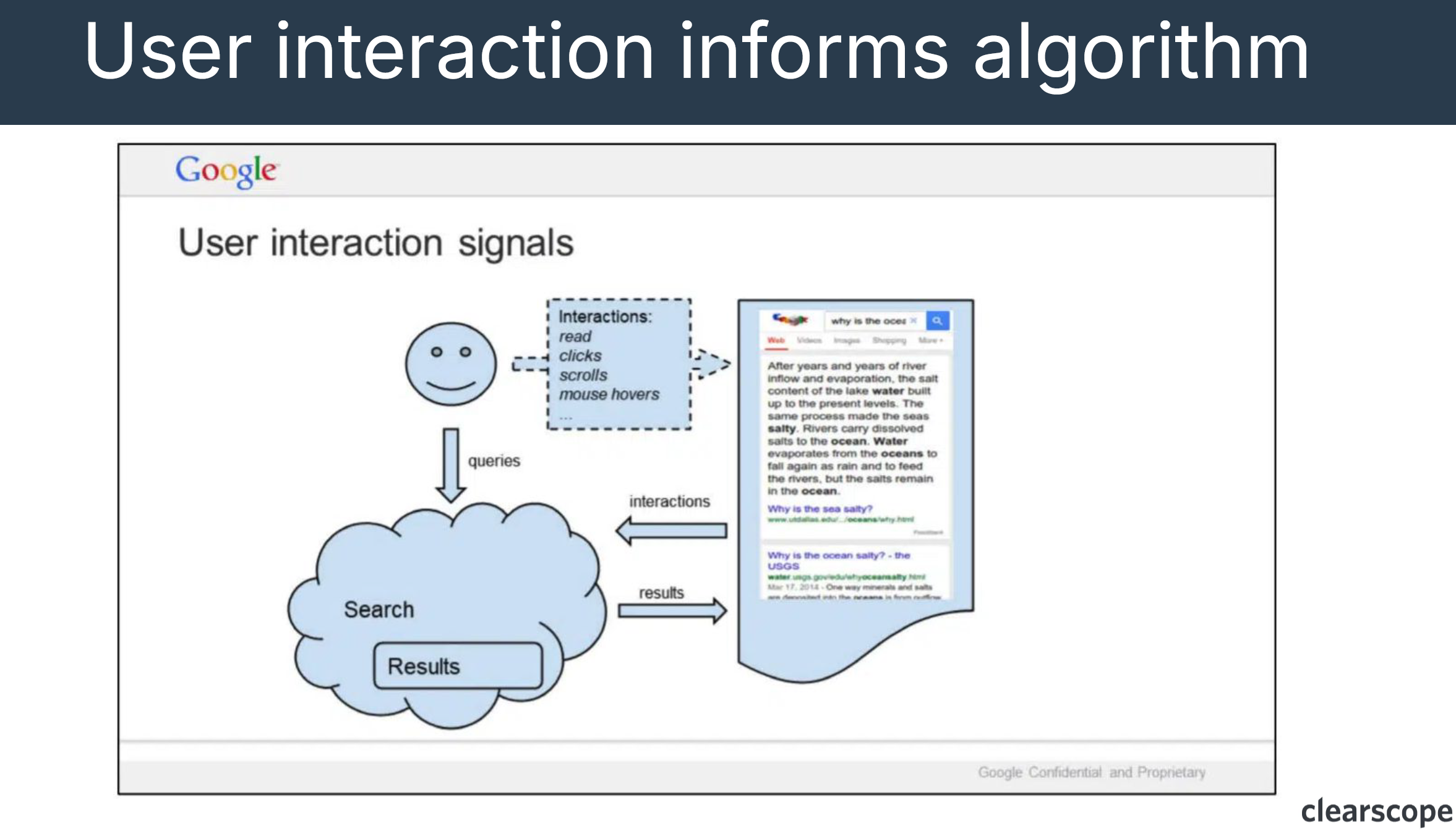
“The interaction then influences the Knowledge Graph. So Google search was about authority and quality content and whoa, okay there’s a new possibility from an entity perspective that Google search can now be linked to this internal document API link. So they’re now making that loose connection because iPullRank qualified to sway Google’s information for the topic in the Knowledge Graph and they’re saying, okay we’ll try it. But then the other sources come into play and this is like Search Engine Land this would be, Kick Point, this would be Clearscope if we were to cover it, and so on, then people come in and this is May 28th like literally one day after huge Google search document leak reveals inner workings of how the search works.
So then the SERP actually goes to the authority sites. If I ask Gemini, what topics are Search Engine Land authoritative in? It’s SEO, PPC, search engine, marketing, industry, news, case studies, and expert opinion. Basically it’s the go-to resource for anyone looking to stay informed about the latest developments in the search marketing world.
That’s a shame because SparkToro and iPullRank were the ones who actually broke the news. But Google’s algorithm doesn’t, unfortunately, work in the way that we would hope that it worked. And this is what happens. The SERP goes to the authority’s site, because Search Engine Land now has published a piece of content.
Google has already started to link Google Search with API leaks, and so it’s already a thing. And the page embeddings for Google algorithm is way closer to what this site looks authoritative in, and Search Engine Land is currently the number one, even though they were one day late to break the news. iPullRank is [ranking at] a measly number 17 now. That kind of sucks. ” Bernard Huang
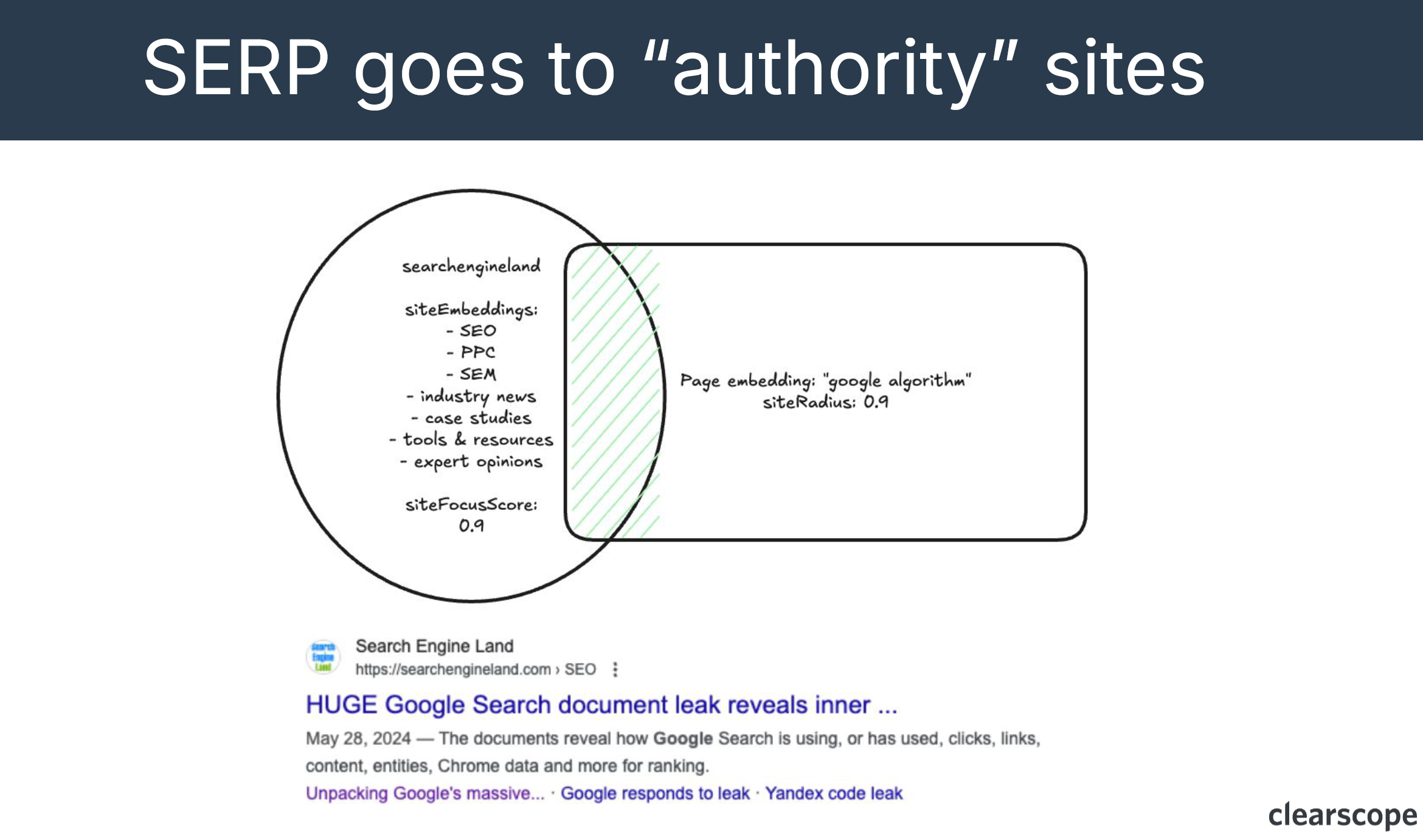
This is why so many small publishers are disgruntled with Google, they go out of their way to provide first-party data, experience, and perspective rich content and then a larger publisher (we’re looking at you Forbes 🙄) comes along and replicates it using semantic SEO entity recognition and the smaller publishers are no longer ranking.
How to understand your own authority on topics
- Identify core topics: Begin by determining the topics Google thinks your site is authoritative in, ask Gemini “what topics are [your company] authoritative in?”
- Analyze Google Search Console data (GSC): Pull query data from your Google Search Console. Export this data into a CSV file, including associated click volumes. Then use a tool like Claude to cluster queries based on relevance and traffic to see what topics drive the most clicks. Check out all of these prompts and instructions from Andy Crestodina if you are new to AI Powered Data Analysis.
- Map authority: Graph the results of your clusters to see where your site has the most authority. Remove your branded queries to better assess topic authority. For example, if “content optimization” or “keyword research” has high captured search volume, this indicates strong authority. The “site embeddings” Bernard refers to are your content’s focus areas based on how well you rank for related searches.
- Calculate captured search volume: Track the search volume for each topic cluster. Look at the average keyword volume for the primary search term of the topic versus your click data to get your match rate or percentage, revealing how much of the search volume you’re capturing for a specific topic. In Bernard’s example for Clearscope, they got 1,137 clicks for Knowledge Panel which had an average monthly search volume of 1,900; 1,137/1,900 = 59.8%.
- Assess Topical Authority: Your authority is directly mapped to how much search you’re capturing for the given topic. Using captured search volume helps you better understand how well your content ranks, you may identify that you are maxed out on a certain topic even if the relative amount of traffic is low.
“[Site radius] influences, directly your content’s ability to A) seed into the search results and B) influence Google’s Knowledge Graph on the topic, which has been phenomenal. That’s why topical authority has been, this is probably the closest I’ve been able to define topical authority.” Bernard Huang
Real world examples of losers
LyricsRoll got decimated by the early helpful content update due to redundancy. Unlike its competitor Genius, which covered all the same entities but provided additional context like producers, related projects, and so on, LyricsRoll offered no unique value beyond basic song lyrics. There is low or no information gained compared to more comprehensive sources like Genius. This redundancy in entity coverage means a loss in rankings.
“Redundancy is not plagiarized text. It is entity coverage.” Bernard Huang
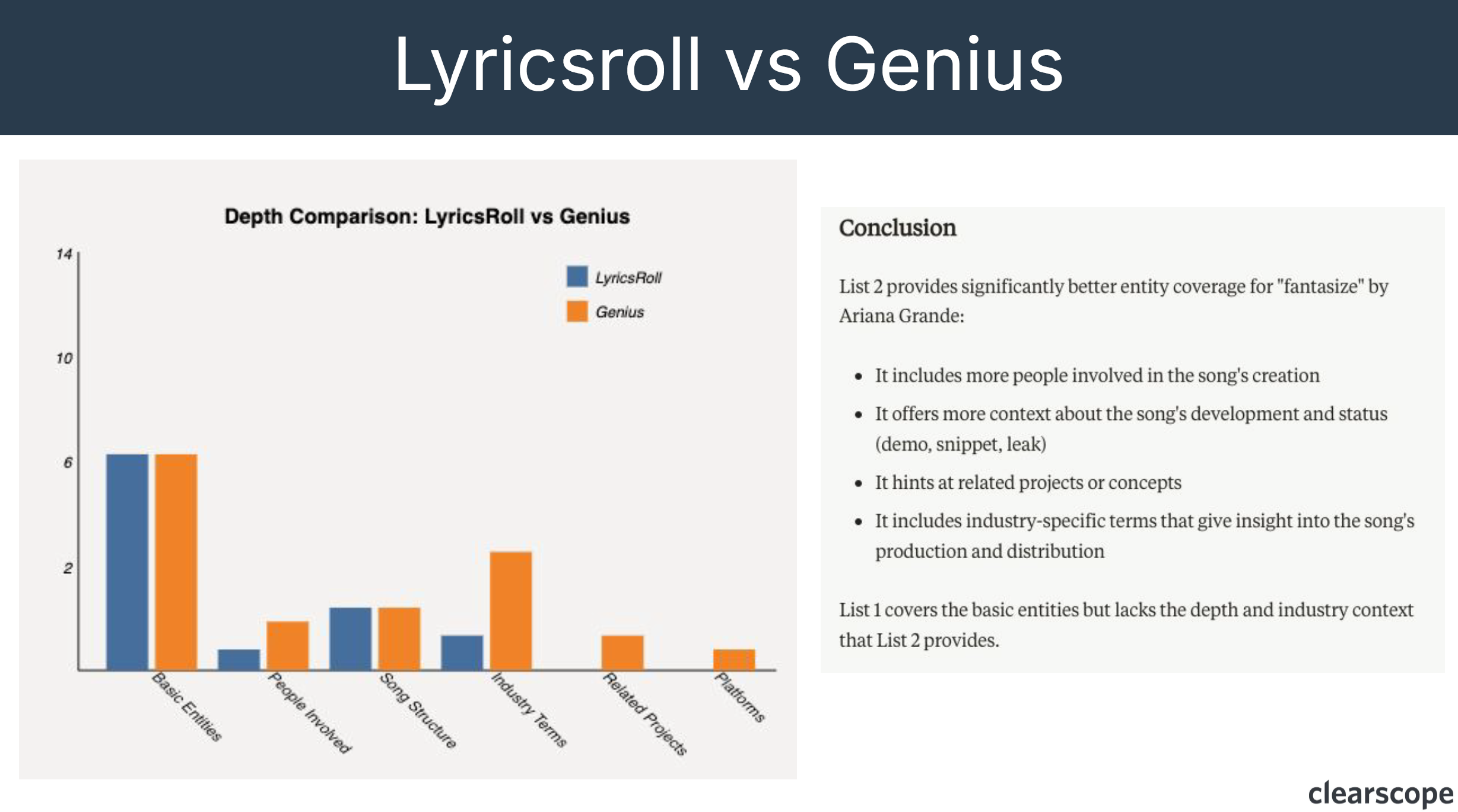
Key factors for ranking
- Matching Google’s knowledge graph: Google uses its Knowledge Graph to assess known entities related to a topic. To rank well, your content must align with these core concepts, as failing to mention them can be a deal breaker. So for example, if you want to be an authority on SEO who ranks, it’s clear that writing about the basics is table stakes. If you do not cover entities like backlinks, internal links, technical SEO, and so on, in Google’s eyes you don’t deserve to rank.
- Providing meaningful information gain: Beyond covering the basics, Google expects you to add value by contributing new information, experiences, or first-party perspectives. This enhances Google’s understanding and boosts your content’s relevance in search results.
“I think there is a basic amount of semantic understanding that Google expects for any given topic, like this. I think from there Google wants you to provide meaning to the topic. In that particular case, I don’t think there is a right or wrong answer here. I think that as long as you match the basic understanding of what the Knowledge Graph is, you can then add whatever you want to add, and just know that is actually important because you’re adding new first party data, experiences, perspectives to Google’s understanding.” Bernard Huang
Start optimizing for information gain
Is your mind spinning now? There was so much information gained here 😉 it’s a lot to take in. If you want to start optimizing for in your area of expertise then according to Bernard you need to:
- Determine where you are in terms of site embeddings and site focus score. Gemini is a great way to understand this as well as using your own data. If you are a new business you will have your work cut out for you. Build links, create great content, slowly inch your way up so that you qualify to be able to contribute to the topical evolution.
- Cover the known entities that Google expects. If you don’t do this, you won’t ever be able to rank or even be considered an authority.
- Inject your own story, perspective, experiences, and first-party data (aka information gain).
❓ Have a question for Bernard on information gain? Feel free to leave a comment on the YouTube video or email them directly to us!